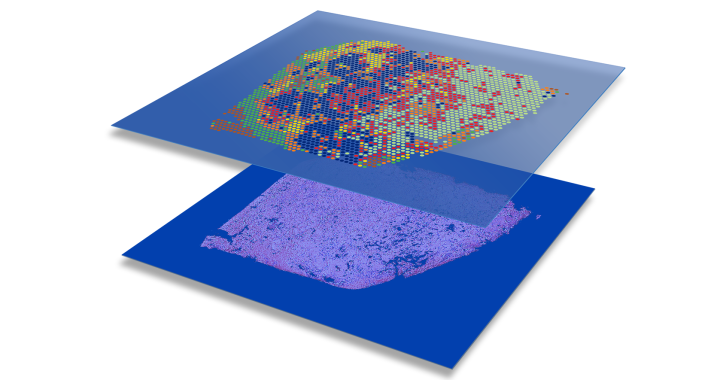
New biological methods are realized with high speed, so it can be difficult to maintain an overview of which techniques are suitable for which purpose. Spatial transcriptomics has myriad potential applications. In this blog, we outline the most important five and direct you to further reading material.
Spatial transcriptomics combines data on the gene expression of cells with information on the transcript source location. This way, you can analyze the transcriptome of cells within their organizational context. How can you apply it?
In two previous blogs, we explain more about spatial transcriptomics in general (read it here) and about the spatial transcriptomics service that we can provide as a pilot, called Visium Spatial Gene Expression (read it here).
Read more: Spatially resolved transcriptomics add a new dimension to genomics [Nature Methods]
1. Create spatial maps of complex tissues
Spatial transcriptomics is ideal for defining and identifying cell (sub-)types and mapping them out on a tissue scale. In this way, it can accurately reveal the spatial organization of cell types in ever more complex tissues. These could be primary tissue, clinical samples such as tumor biopsies, or organoids. Researchers already utilize spatial transcriptomics to generate maps of e.g. the human cornea, kidney, and even the brain.
Read an example: A multimodal cell census and atlas of the mammalian primary motor cortex [Nature]
2. Reconstruct cell development pathways
Single-cell transcriptomics enables you to identify the differentiation state of a cell and so reconstruct the cell development pathway. Going one step further, spatial transcriptomics can combine this with spatial data to reconstruct more complex developmental processes such as embryonic development, tissue regeneration, and stem cell dynamics. In combination with drug assays, spatial transcriptomics applications visualize how tissues change in reaction to specific treatments.
3. Identify drug targets
Proteins inside and on the surface of cells are often-used drug targets, and you can identify many of these targets by their transcripts using single-cell transcriptomics. You can use spatial transcriptomics to map out these drug targets in space. In treating complex disease tissue, such as infected tissue or heterogenic tumors, this can be crucial for e.g. selecting combination therapies or estimating chances of drug resistance and disease relapse.
4. Immune profiling
In a similar fashion, single-cell transcriptomics can be combined with immune profiling to identify specific immune cells (like B- and T-cell subsets). Adding the dimension of space, spatial transcriptomics can be applied to map the repertoire of immune cells in tissues. Measurements in homeostasis, during an immune response or as a result of treatment, can provide answers to fundamental immunological questions. It can also inform therapy choice, for example, by mapping immune cells in a tumor microenvironment.
Read more: The use of single-cell multi-omics in immuno-oncology [Nature Communications]
5. Machine learning
Finally, spatial transcriptomics can aid optimization in computational biology. By combining spatial transcriptomics data with other spatial data, such as microscopic images or spatial proteomics data, you can use it as a dataset for machine learning. As such, you can improve the computational power of methods that measure e.g. cell morphology or protein regulation.
Read an example: Integrating spatial gene expression and breast tumour morphology via deep learning [Nature Biomedical Engineering]